1. The 3Rs principles are making a difference in preclinical research
Over the last few decades, the systematic promotion and use of the 3Rs principles have replaced many animal experiments, reduced the number of animals used and kept their stress to a minimum. These efforts are being consistently pursued because it is not currently possible in all cases to dispense with animal experiments for the research and development of new active substances. This is the case, for instance, when complex organs such as the brain or the interaction between different organs are being investigated. Animal experiments are also used to research whether a potential new active substance is effective against a pathogen or tumours, for instance, and to establish the maximum dosage with which it can be safely applied. These animal experiments, also referred to as the preclinical phase, are required by law. Only when its safe and effective use in animals has been demonstrated may an active substance be tested on humans in clinical trials.
Successful alternatives to animal experiments
Nowadays, a whole range of alternative methods are used instead of animal experiments, such as human cell and tissue culture. And “organs-on-a-chip”, based on three-dimensional cell systems, offer new opportunities to minimise the number of animal experiments needed. Today, several organs can be accommodated on a chip the size of a USB stick and combined with each other to model the dynamics of a human organism. Using organoids obtained from patients, it is also possible to emulate key aspects of human organs, for instance parts of the gut, outside a living organism, thereby predicting adverse effects of potential medicines in human bodies. These groundbreaking developments may lead to a further reduction in animal experiments. However, these animal-free alternatives will only be recognised if their results are just as reliable or better than those of animal experiments.
Recognition for alternative methods is crucial
The time-consuming review and validation of alternative methods means it may take years or even decades before such methods are recognised by the regulatory authorities as a replacement for current animal experiments. Several institutions have been created at an international level in order to make headway with the validation of these alternatives to animal experiments. For instance, these include the European Centre for the Validation of Alternative Methods (ECVAM) and, in particular, the International Conference on Harmonisation of Technical Requirements for Registration of Pharmaceuticals for Human Use (ICH). The ICH is promoting the global standardisation of accreditation criteria for medicines, and is also making a substantial contribution to the recognition of alternatives to animal experiments by the relevant regulatory authorities in Asia, Europe and the USA.
2. Harnessing the potential of big data and artificial intelligence for the 3Rs in preclinical research
Utilising existing and future data
The recognition of alternative methods is one thing. But what opportunities does big data offer for adding extra impetus to the 3Rs? Big data has as yet untapped potential to accelerate and further improve biomedical research. But how would it work? Research findings are published in scientific journals, as are the results of animal experiments. But the study data – for example information about the relevant methodology, findings, from what dose a medicine caused side effects or when a medicine is effective against a disease – is not recorded anywhere in such a way that researchers can use and compare the results of previous studies. To learn from animal experiments that have already been carried out, the available test data would have to be collected via a digital platform, for instance, and linked in such a way that researchers could quickly and easily access the information – regardless of whether the results were positive or negative. For example, tablets consist of an active substance that is effective against a disease, and also substances to bulk out the tablets and allow them to be compressed. These bulking agents must not trigger any toxic effects, and so also have to be tested using animal experiments. Since the bulking agents are widely used in tablet manufacturing, it would be useful to record all toxicological tests in a database so that other researchers at universities or in pharmaceutical companies that conduct research could use these test results for their own applications, without having to repeat the tests. Big data is therefore only relevant if data can be linked and made accessible. Making this data collectively usable also necessitates the initiation of a cultural change and the breakdown of existing silos within institutions and between research institutions and companies.
The use of artificial intelligence requires large datasets
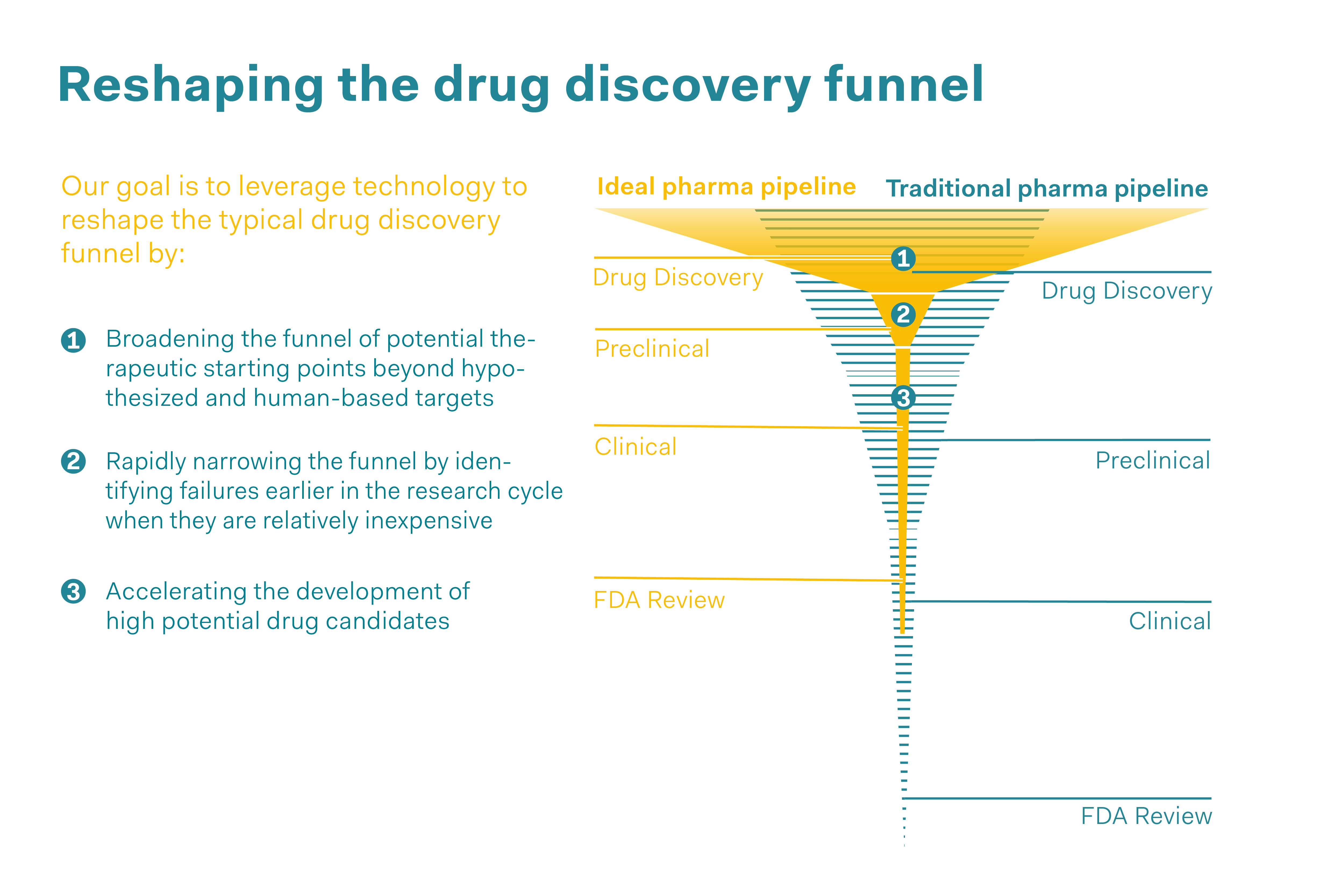
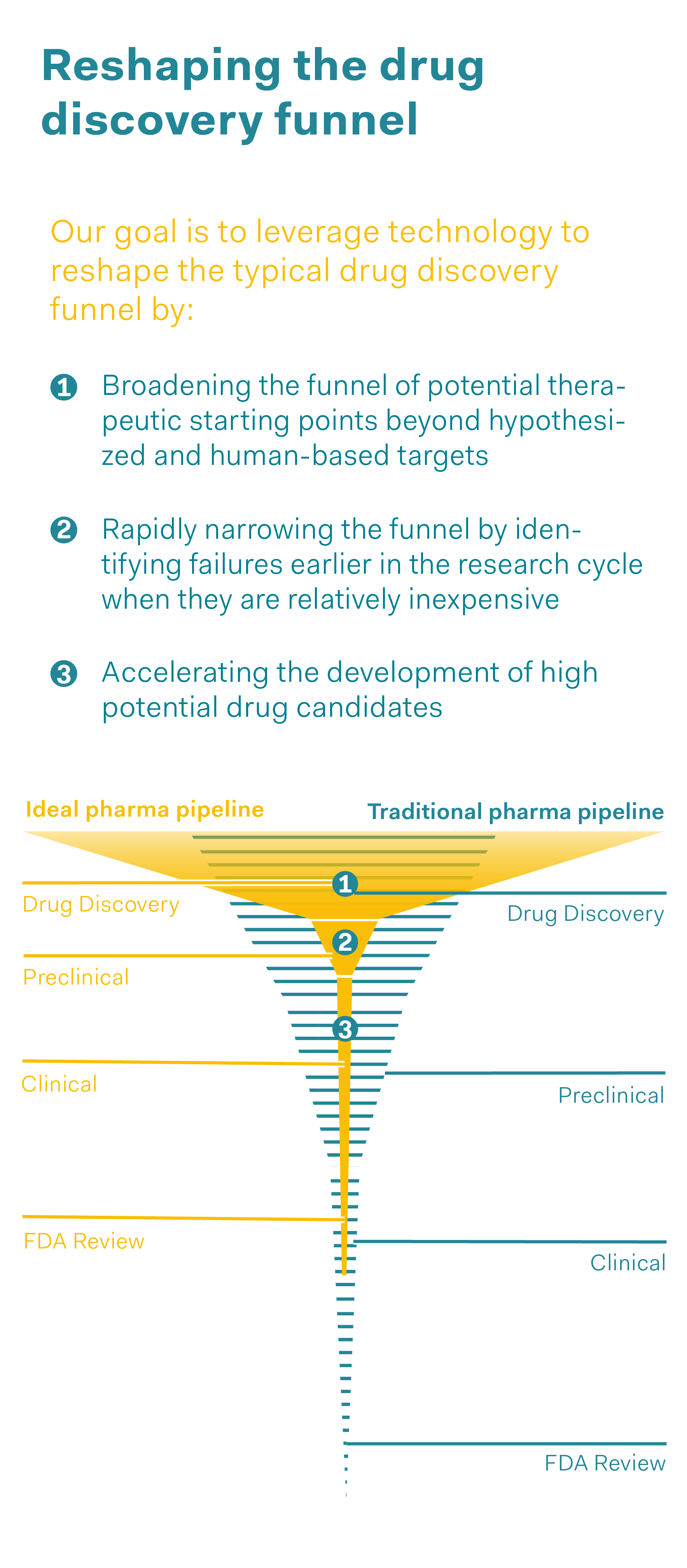
If large volumes of data are available, the question is then whether artificial intelligence (AI) should be used. In fact, linking animal experiment data could not only result in these experiments not being replicated, but also in entirely new techniques and applications being developed. For instance, artificial intelligence could be used to model animal experiments and predict whether a medicine would be effective or how safe it would be, without the need for animal studies. In conventional drug development, “drug discovery”, i.e., the phase in which the new active substance is discovered and tested “in vitro” (e.g., in cell cultures), makes up the largest proportion of the development process (in terms of the number of tests conducted on potential candidate substances). The next stage is to test a potential medicinal product using animal experiments, before it is used on humans in clinical trials. Artificial intelligence could play a crucial role in expanding this “in vitro” research and making some of the animal experiments in the preclinical phase obsolete, in other words, further reducing them. AI also has the potential to speed up the process of drug development from the concept through to approval, and to discover errors in an earlier phase of development so that only highly promising potential active substances get as far as the clinical phase. However, using artificial intelligence presupposes a large volume of data and access to this data, while also ensuring that the protection of intellectual property is taken into account. Above all, it requires researchers with the expertise to use AI in a targeted manner, evaluate the findings obtained and make decisions based on these findings.
3. First steps taken towards data networking
The industry is making a pioneering contribution towards reducing animal experiments
In a highly regarded project, four pharmaceutical companies have joined forces with support from the European Federation of Pharmaceutical Industries and Associations (EFPIA) to launch a voluntary, non-profit initiative for the provision of data by the industry, in collaboration with the European Chemicals Agency (ECHA). The aim is to make high-quality, previously unpublished physical-chemical, toxicological and ecotoxicological substance data publicly available from the companies’ archives. Thanks to the extended access to chemical safety data, the effectiveness of database tools for predicting the properties of chemical substances is being improved. This data can also be used by experts from academia and research-based pharmaceutical companies to create models in order to gradually reduce or completely eliminate animal experiments for chemical substances. The ECHA has already agreed to support this initiative, act as a broker and provide a neutral platform for disseminating this data. A test phase is currently underway, in which the ECHA, EFPIA, Boehringer Ingelheim, F. Hoffmann-La Roche, Johnson & Johnson and Merck KGaA are taking part. The aim is to create a programme in which additional companies can participate and make their archive data available.
Advancing the 3Rs with data platforms
These days, complex datasets in pharmaceutical companies are often captured, stored and jointly used in the form of spreadsheets. AstraZeneca’s PreDICT (Preclinical Data Integration and Capture Tool) platform was developed in collaboration with the data analysis firm Tessella. This involved first of all defining a series of data standards with which in vivo study data from all areas of research can be represented clearly and comprehensively. Preclinical data was captured and analysed, including pharmacokinetic data (this data describes all the processes to which a medicinal substance is subjected in the body, such as the absorption of the substance, its distribution within the body and its biochemical modification and decomposition), pharmacodynamic data (data on adverse effects and the correct dosage in order to achieve the desired effect within the body), as well as efficacy data. The system ensures data integrity and enables scientists to rapidly find, integrate and jointly use in vivo datasets to predict optimal dosages and schedules in clinical trials. As well as saving time, reducing outsourcing costs and simplifying workflows, a significant improvement in data quality and increased confidence in the data were observed. This rapid access to high-quality data makes it significantly easier for researchers to develop models for the behaviour of drugs in real organisms. The predictions produced by these models are also intended to completely replace some in vivo experiments, or to obtain the maximum findings per animal and reduce the number of animals used.
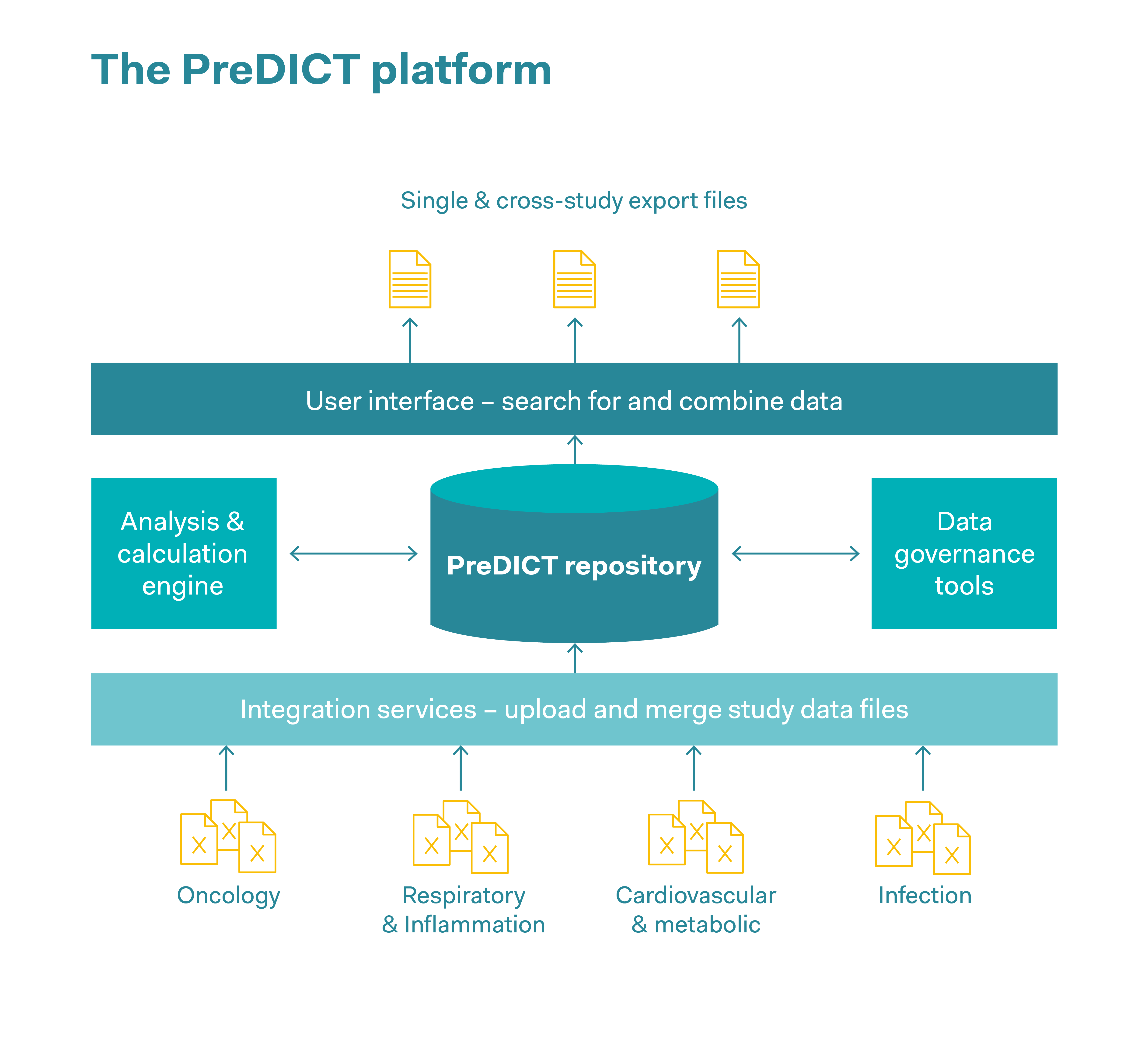
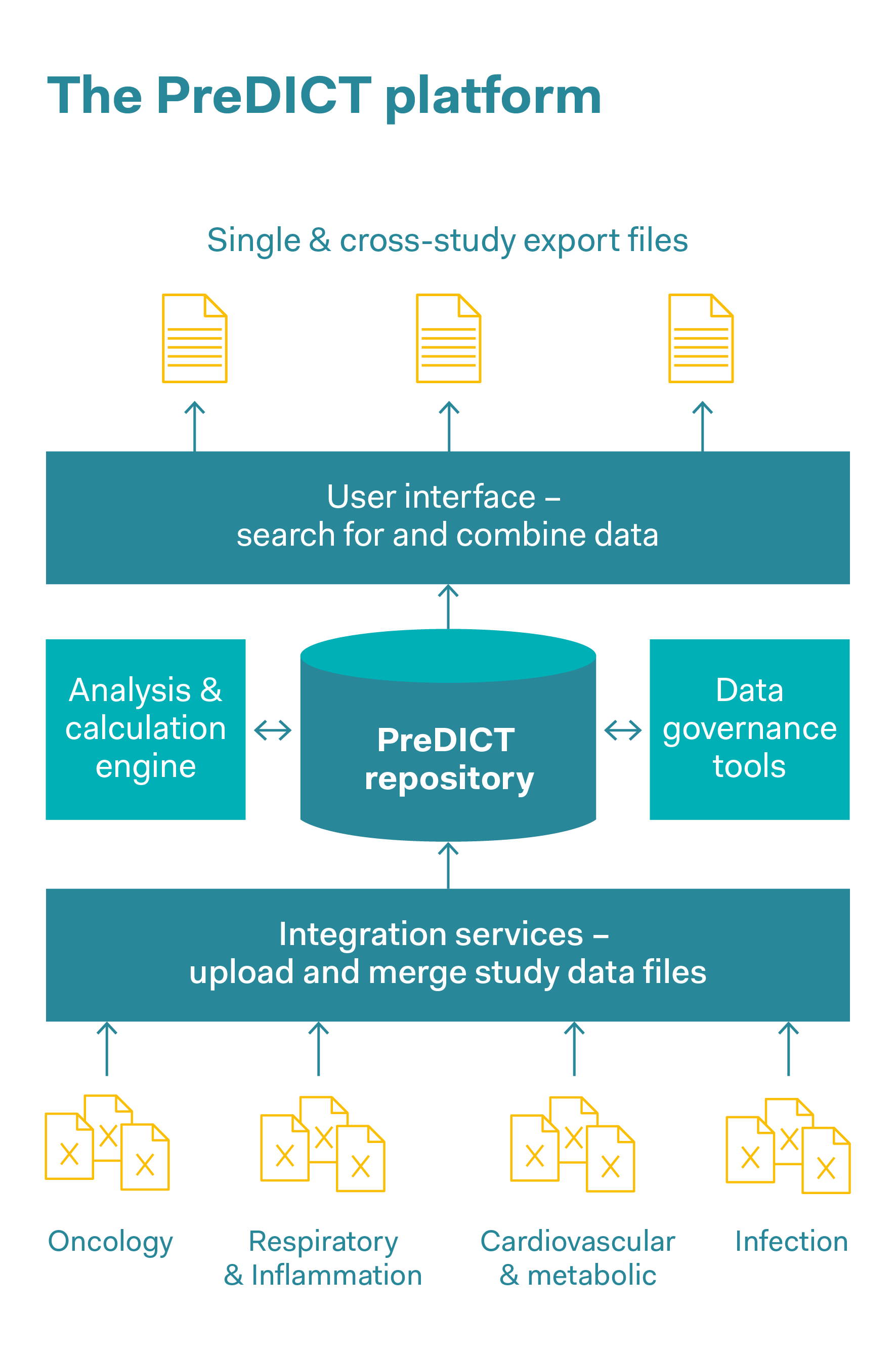
Reduce
The ability to access a large amount of data helps researchers to optimise how they design experiments so that the maximum findings can be obtained for each animal used. Access to archived data helps to avoid in vivo experiments that have already been carried out and reduce the number of animals used in new studies, for example by reusing control group data from earlier comparable studies.
Replace
Rapid access to high-quality data makes it easier for researchers to model the behaviour of active substances in real organisms. The better the in vivo data is maintained, the more likely it is that reliable model predictions will be made that could replace in vivo experiments.
Refine
The database makes it possible to combine datasets from many studies to form a meta-analysis, and provides a comprehensive insight into the way animals are used. This helps to refine in vivo experiments.
Using big data to improve research and patient safety
Supported by various pharmaceutical companies and academic partners, technology company GMV has created a biomedical data technology platform as part of the eTRANSAFE project. This platform is intended to improve the development of new drugs and make them safer for patients. The overarching goal of eTRANSAFE was to drastically improve the predictability, feasibility and reliability of safety assessments during the drug development process. This was achieved through the development of the eTRANSAFE ToxHub platform, which brings together preclinical and clinical databases in an integrative data infrastructure, combined with innovative computational and visualisation tools. This generated a sufficiently large quantity of biomedical data in order to draw conclusions using big-data technology. The benefits of the project include more efficient studies, shorter research times and better toxicity results. Part of the project compares preclinical and clinical studies to better predict what happens in humans in the clinical phase.
eTRANSAFE – an Innovative Medicines Initiative project
eTRANSAFE was developed as part of the «Innovative Medicines Initiative (IMI)», Europe’s largest public-private initiative, which is supported by the European Union’s Horizon 2020 research and innovation programme and the European Federation of Pharmaceutical Industries and Associations (EFPIA). The project aimed to make a large quantity of preclinical and clinical data available, while adhering to data governance procedures. One prerequisite was the availability of relevant, high-quality datasets. But in order to use this data optimally, major challenges had to be overcome, such as encouraging the exchange of information between competing organisations and fostering the appropriate control, standardisation and annotation of data quality.
4. Jointly tackling cultural change for the 3Rs using big data
The examples mentioned typify the commitment of the pharmaceutical industry to break new ground, share data and make it available to researchers, and make increased use of the opportunities of big data and artificial intelligence, even in preclinical research. This could be the start of a new chapter enabling further significant progress to be achieved with the 3Rs. Discoveries in basic medical research also benefit patients. For example, digital methods were and still are being used to research the structures and functionality of the coronavirus.
It is important that the opportunities presented by digitalisation, which is widely used in society, are also increasingly used in drug research. But this will only happen if preclinical research data is made widely available.
Interpharma aims to promote the dialogue on data networking in the preclinical field and help to advance this important subject in Switzerland and in an international context. Cultural change, collaboration between all researchers and appropriate political measures (recognition of new alternative methods and artificial intelligence) as well as the protection of intellectual property are vitally important if the potential of big data and artificial intelligence is to be exploited in order to replace animal experiments in developing drugs and treatments.
5. What the experts say
Interpharma aims to promote the dialogue on data networking in the preclinical field and help to advance this important subject in Switzerland and in an international context.